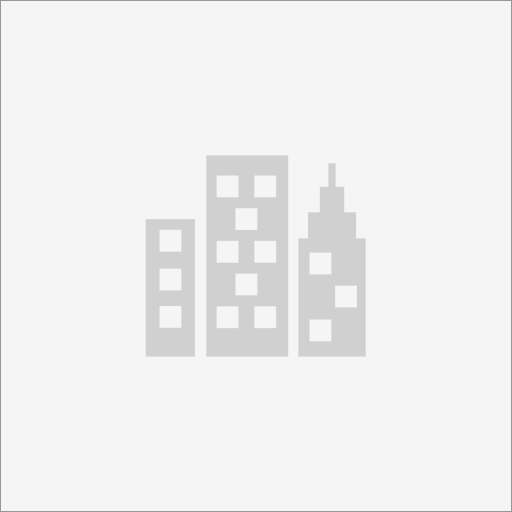
Mines Paris PSL Université
A doctoral position is open within the Center for Robotics, Ecole des Mines, PSL University, in the
framework of the PIA4 ReSource project « Conservatoire des Gestes et Savoir-faire des Métiers
d’art et de Fabrication », coordinated by the Manufacture des Gobelins.
Context: Motor performance as well as the acquisition of motor skills in arts, sports, or any other
professional field (such as craft professions) requiring a high-level manual mastery is correlated with
a large number of human factors. First of all, motor performance is related to the subject and his/her
anthropomorphic characteristics, experience, emotional and physical state (intrinsic human factors)
as well as to his/her environment, workspace, rhythm of work, the protocols and the rules that the
subject must follow (extrinsic human factors). However the effect of these Human Factors (HF) vary
depending on the level of expertise. For example, it is expected that stress, the level of
concentration or fatigue, or other external stimuli, impact the motion variables that are used to
evaluate the performance in a different way on medium or advanced craftsmen.
The analysis and evaluation of motor performance can be useful for various reasons, such as its
better understanding, preservation, identification of ergonomic issues, improvement of
manufacturing processes and especially training/transmission of know-how. It is therefore
necessary to define which are the important aspects to be evaluated through a computational
analysis approach based on the definition of motor performance indicators (MPI). Existing MPIs
mostly focus on biomechanical aspects without necessarily reflecting the effect of human factors
on performance. While their role during the execution of gestures is essential. They impact not only
the performance itself but also the reception of sensory information, its processing, its assimilation
and the permanent change in the resulting motor patterns (learning).
Nowadays, motion capture technologies (inertial sensors, RGB-D camera etc.) and humancentered
artificial intelligence algorithms make possible to record a very rich and complete set of
data/signals and to model them. In these signals are encoded not only the correlations but also the
causal relationships that exist between the different factors and performance indicators.
Scientific objective: In this context one of ReSource project goals is to propose a methodology to
study the effect of HF on MPI applied to various level of manual expertise, to analyse and visually
represent the findings. The aim is to analyse human motion data collected from different craft (gold
smithery, jewelry etc.) experts when performing dexterous gestures/actions/postures and to identify
and evaluate a certain number of relevant MPI, to explore their correlations with HF, to propose
new ones that encode the best these correlations and also to visualize them.
More precisely the Phd candidate will work with human motion data recorded with motion capture
systems (such as a full body IMU suit) of experimented craft experts. These datasets (timeseries)
contain an important number of variables which will be used to measure and define the MPIs that
encode and reflect the best the dexterity, the inter and intra personal similarities and differences in
the way to perform the gestures. The Phd candidate will be also expected to propose new MPI
related to craft manual expertise that incorporate and correlate the motor performance to important
aspects in creative manufacturing such as quality, productivity, creativity etc.
After having defined the most relevant MPI the Phd student will explore their relation
(correlation/spurious correlation/causality/causation/hidden confounders etc.) to a set of HF by
using causal AI methods. These methods (score based, constraint-based, causal deep learning
etc.) permit to perform causal discovery upon observational data while putting the accent on the
explainability and interpretability of the model built. The result of this modeling is expected to
contribute to a better understanding of certain HFs effect on MPI in the context of creative manual
manufacturing professions.
The student will also propose a visual representation strategy of the MPI and of the correlations
identified that could be based on causal graphs or causal maps and that would easily visually reveal
important factors for observational learning. A serie of experiments will be conducted by the
candidate to evaluate whether the visual representation is effective and suggestive , whether it fills
the gap between the findings and their communication to the final user, between the complexity of
the information and of the display.
References
Newell, K. M. (1987). Skill learning and human factors: a brief overview. Ergonomics and human
factors: Recent research, 95-102.!
Proctor, R. W., & Van Zandt, T. (2018). Human factors in simple and complex systems. CRC press.
« Skill acquisition in Sport: Reserach, theory and practice » Edited by Nicola J. Hodges, A. Mark
Williams, 2020
« Causal Deep Learning » by Jeroen Berrevoets, Krzysztof Kacprzyk,Zhaozhi Qian,Mihaela van der
Schaar, ArXiv
Manitsaris, S., Senteri, G., Makrygiannis, D., & Glushkova, A. (2020). Human movement
representation on multivariate time series for recognition of professional gestures and forecasting
their trajectories. Frontiers in Robotics and AI, 7, 80.
Chen, S., Li, J., Andrienko, G., Andrienko, N., Wang, Y., Nguyen, P. H., & Turkay, C. (2018).
Supporting story synthesis: Bridging the gap between visual analytics and storytelling. IEEE
transactions on visualization and computer graphics, 26(7), 2499-2516.
Ullen, F., Hambrick, D. Z., & Mosing, M. A. (2016). Rethinking expertise: A multifactorial gene–
environment interaction model of expert performance. Psychological bulletin, 142(4), 427.
Possible extensions of the thesis :
How do these visual representation effect observational learning? Which indicators are the most
interesting for in the context of observational learning?
In observational learning the subject is passive and is observing details of expert performance in
order to build mental representation of the expert movements. Observational learning gives the
possibility to the learner to conduct additional processing, based on the analysis, visualisation,
annotation etc. of expert’s data and to mentally embody and decode various motor components of
the expertise.